Understanding Artificial Intelligence: A Clear Definition
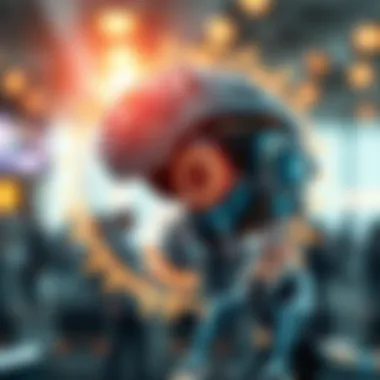
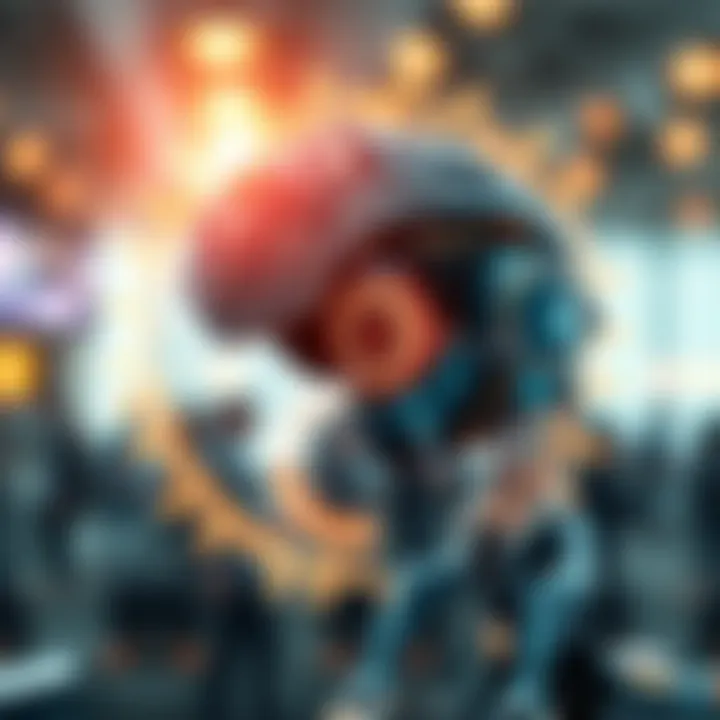
Intro
Artificial Intelligence (AI) isn’t just a buzzword thrown around at coffee breaks. It’s a transformative force that’s shaping our modern world. As we peek behind the curtain of this fascinating technology, it becomes crucial to clarify what AI really means. Our ultimate goal is to break down the complex jargon and help you grasp the specifics and nuances of AI without needing a PhD in computer science.
AI, at its core, refers to the capability of a machine to imitate intelligent human behavior. In simpler terms, it's about machines making decisions, learning from data, and even performing tasks previously reserved for humans. But don't let the term fool you; AI isn’t some magical black box. It runs on algorithms and patterns derived from extensive amounts of data, making it quite relatable if you look at it through the lens of using a search engine or recommendations on streaming platforms.
To tackle this concept effectively, we’ll explore various dimensions of AI, including its fundamental principles, types, and the impact it has on today’s technology landscape. This breakdown will clear up confusion and shed light on the essence of artificial intelligence, paving the way for a more informed understanding.
In the digital age, understanding AI isn't just a luxury—it’s a necessity. Whether you’re an investor looking at AI-driven companies, a student eager to learn about future technologies, or a financial advisor aiming to guide clients in the right directions, a solid grasp of AI concepts will serve you well in navigating the new frontier of technology.
Prologue to Artificial Intelligence
Artificial Intelligence (AI) is not just a buzzword tossed around in tech circles; it’s an essential concept shaping modern life across various sectors. Understanding AI is like holding a mirror to the unfolding future of technology. As it seeps into daily routines—from chatbots in customer service to predictive algorithms in finance—we must grasp its essence to leverage its benefits.
The importance of diving into AI lies in the fact that it’s transforming industries. For example, in healthcare, AI is improving diagnostic accuracy, and in finance, it’s analyzing vast datasets to identify smart investment opportunities. By knowing what AI is, its history, and how it functions, individuals stand to make informed decisions—be it as investors or as everyday consumers navigating the tech landscape.
An introductory comprehension of AI promotes awareness around its potential, and it allows one to understand the considerations it raises, be they ethical or practical. Certainly, while AI offers immense advantages, recognizing its limits and the implications of its use is equally crucial. Striking this balance prepares everyone to engage with the evolving conversation of AI.
Defining Artificial Intelligence
So, what exactly is Artificial Intelligence? At its core, AI refers to the capacity of machines to mimic human cognitive functions like learning, reasoning, problem-solving, and understanding language. Picture a mind—AI tries to recreate it in a software framework.
The term was coined in the mid-20th century, but its foundations can be traced back even further. AI systems, as they stand today, come in various forms, designed for specific tasks—what we might call "narrow AI." These specific systems are capable of performing suitably complex functions but cannot operate beyond their programmed capabilities.
Important to note: AI does not equate to sentience. As of now, AI lacks personal experiences, emotions, or consciousness. It operates based on data input and programmed algorithms. In short, when we talk about AI, we’re usually referring to algorithms and models that interpret data and produce actionable insights.
For instance, when we use Google Maps, AI processes real-time traffic data to navigate our routes efficiently. This kind of functionality typifies what many people experience as "AI" in their daily lives without necessarily recognizing the underlying technology at play.
Historical Context of AI Development
The journey of artificial intelligence is as fascinating as the technology itself. The roots of AI stretch back to ancient times—from myths of intelligent automatons to philosophical inquiries on mind and matter. However, modern AI began forging ahead in the mid-20th century.
In the 1950s, pioneering thinkers like Alan Turing laid the groundwork with the famous Turing Test, which proposed a measure of a machine's ability to exhibit intelligent behavior equivalent to that of a human. Shortly thereafter, the Dartmouth Conference in 1956 marked the birth of AI as a field of study, where scholars came together to discuss and explore the potential of machines to learn and reason.
As the decades rolled on, AI encountered waves of excitement and discouragement, often referred to as "AI winters" during periods of funding cuts and waning interest. Yet, breakthroughs continued—input advancements in computing power and availability of data saw AI begin to flourish in the 21st century, spurring developments like deep learning, neural networks, and natural language processing.
"The dream of artificial intelligence is not a mirage; it's a journey with a winding roadmap marked by collaboration and innovation."
Understanding this historical context enriches our grasp of AI. It highlights the persistence of the human imagination in striving to make machines more capable and intelligent. Knowing how far AI has come gives us insight into where it might lead us in the future.
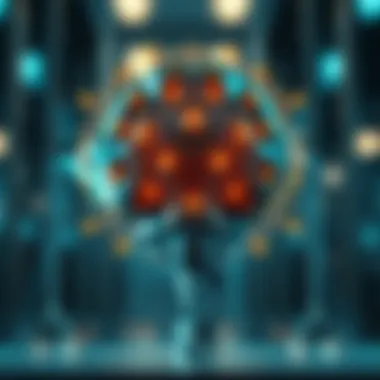
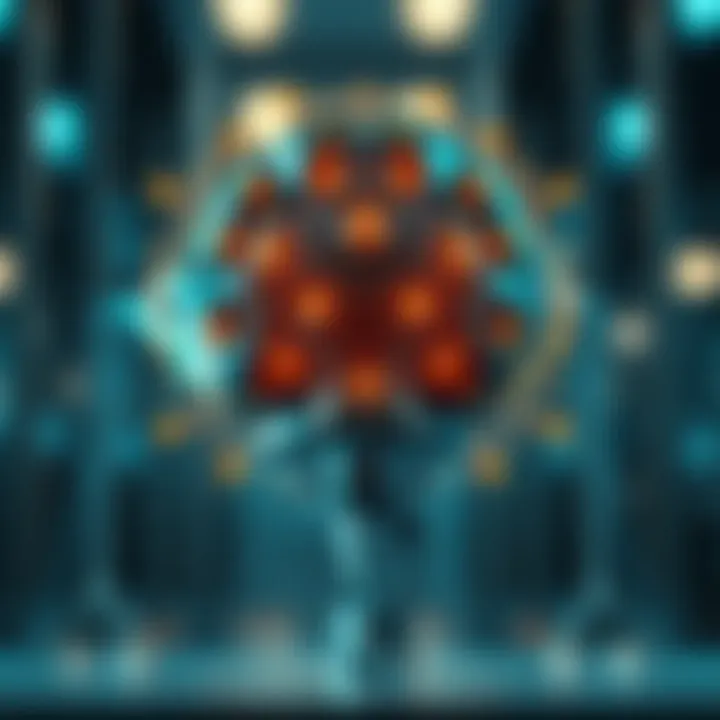
Core Principles of Artificial Intelligence
The essence of artificial intelligence hinges on several core principles that guide its development and application. These principles are vital as they lay the groundwork for how AI systems learn, adapt, and function in various domains. By understanding them, stakeholders—ranging from investors to educators—can better appreciate how AI can revolutionize different industries. This section digs into the heart of AI, exploring critical elements that underpin its functionality.
Understanding Machine Learning
Machine learning serves as the backbone of many AI systems, differentiating intelligent algorithms from traditional programming. Essentially, it’s about allowing machines to learn from data without explicit human intervention. Imagine training a dog; you show it a trick several times, and over time it understands what you want. Similarly, machine learning involves feeding the algorithm with ample data, and through various techniques, it learns to make decisions or predictions based on that data.
There are a few key types of machine learning:
- Supervised Learning: Here, algorithms learn using labeled datasets. Think of it as a teacher guiding students by providing answers. An example could be predicting housing prices based on features like the number of bedrooms, location, and square footage.
- Unsupervised Learning: In this scenario, algorithms find patterns within unstructured data. It’s akin to exploring a new city without a map; they identify clusters or groupings. Customer segmentation in marketing is a common application here.
- Reinforcement Learning: This method teaches systems to make decisions through trial and error, akin to rewarding a child for good behavior. A practical example could be training a robot to navigate a maze.
By understanding these types, investors and analysts can identify which machine learning approach suits specific business problems, thus guiding their decision-making in AI investments.
The Role of Data in AI
Data is the lifeblood of artificial intelligence. Without it, AI systems would be as good as a car without fuel—ineffective and unproductive. High-quality data enables AI to provide accurate predictions and insights, making it central to the successful deployment of AI solutions.
A few important aspects of data in the context of AI include:
- Quality Over Quantity: It’s not just about having massive amounts of data; the data must be relevant and accurate. Garbage in, garbage out, as the saying goes. If the input data is flawed, the AI’s output will be, too.
- Diversity of Data: To ensure that AI systems are fair and effective across different demographics, it’s essential to use diverse datasets. This helps in minimizing bias and makes AI applications more reliable.
- Real-Time Processing: In many scenarios, AI needs to work with real-time data to make timely decisions, such as detecting fraud in credit card transactions instantly. This capability is crucial for applications that thrive on immediacy.
By understanding the pivotal role of data, stakeholders can appreciate the intricate dance between data and AI, thereby harnessing its full potential in various sectors, from finance to healthcare.
Types of Artificial Intelligence
Understanding the different types of artificial intelligence is crucial for anyone navigating today's technology-driven world. Each type represents a level of capability and applicability that can impact various industries, ensuring that AI integrates seamlessly into everyday functions. It's not merely academic; the distinctions among these types can dictate how businesses strategize and evolve in a competitive landscape.
Narrow AI vs. General AI
Narrow AI, often referred to as weak AI, is designed to perform a specific task. Think about the algorithms used in face recognition systems or recommendation engines that suggest what to watch next on streaming services. These systems operate under a narrow set of limitations and cannot function outside of their designated roles. For instance, an AI trained to optimize supply chain logistics cannot suddenly draft a novel or negotiate a business deal.
In contrast, General AI represents a theoretical form of intelligence, one that exhibits human-like cognitive functions across a broad range of tasks. This kind of AI would not only excel in tasks similar to Narrow AI's functions but would also develop the capacity to understand, learn, and apply knowledge across multiple domains. However, as of now, General AI remains a concept; we haven't cracked that nut yet.
Reactive Machines and Limited Memory
Diving deeper, we encounter two subtypes within Narrow AI: reactive machines and limited memory AI. Reactive machines do not possess memory and are unable to use past experiences to inform current decisions. A prime example here is IBM's Deep Blue, which famously defeated chess champion Garry Kasparov. It analyzed positions on the chessboard and made decisions based solely on the current state, not on prior matches or strategies.
Limited memory AI, however, steps it up a notch. This type can utilize historical data to inform its decisions. Autonomous vehicles are a perfect illustration of this type of AI. They constantly gather data from their surroundings, learning from past interactions to navigate texture and predict pedestrian movements more effectively. Their efficiency relies heavily on the ability to adapt based on prior experiences.
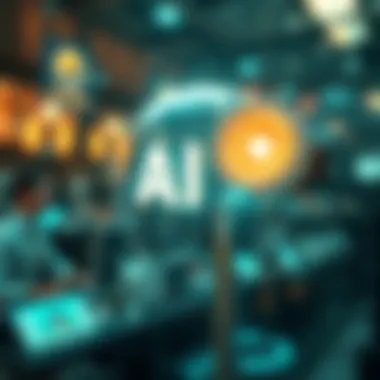
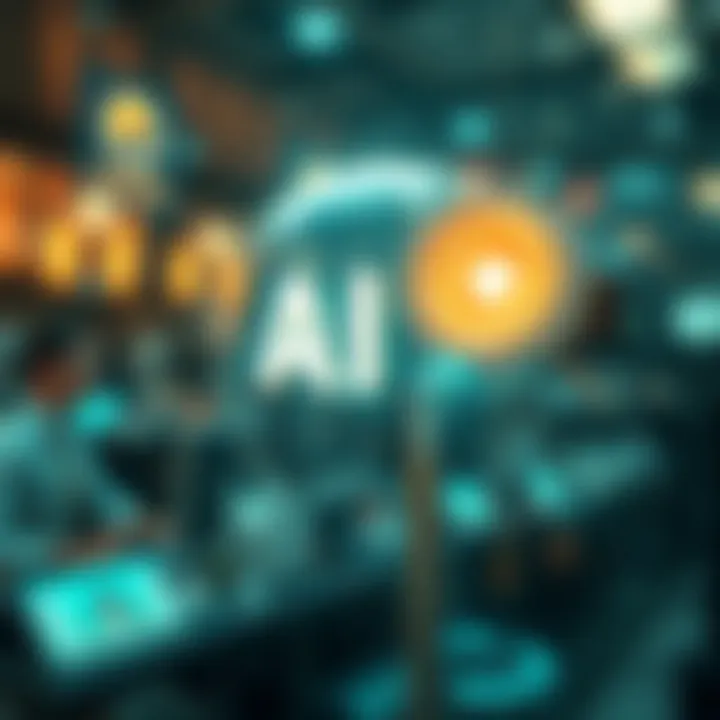
Theory of Mind and Self-Aware AI
While the previous types focus on functionality, theory of mind and self-aware AI represent the future, albeit uncertain, of AI development. Theory of mind AI, which is still in conceptual stages, implies machines would be able to understand human emotions and social cues. Imagine a smart assistant that recognizes when you’re frustrated and adjusts its responses accordingly. This technology could revolutionize customer service and personal assistance.
Self-aware AI goes a step further. This hypothetical AI would possess self-awareness and consciousness, leading to ethical territories we haven't ventured into. If machines could truly understand their existence, the implications would be profound, raising questions about rights, responsibilities, and the nature of consciousness itself.
Understanding these distinctions within AI is imperative for investors and analysts alike. As technology continues to evolve, being informed about the different types of artificial intelligence can help in making strategic decisions and adapting to the rapidly changing landscape. This knowledge is not just valuable; it may soon become essential in your toolkit for navigating the future of work.
"Keeping up with AI means staying one step ahead in an ever-evolving tech landscape!"
By grasping the differences among Narrow AI, General AI, Reactive, Limited Memory, Theory of Mind, and Self-Aware AI, stakeholders can anticipate the opportunities and challenges that lie ahead. There are many layers to peel back, offering fertile ground for exploration and innovation.
Applications of Artificial Intelligence
The impact of artificial intelligence spans a myriad of domains, showcasing its versatility and capability in solving complex problems. Understanding these applications not only illuminates the technology’s current environment but also augments economic growth and societal advancements. When one thinks about AI being integrated into everyday life, the mind wanders through an array of industries where its contributions are most pronounced. Below, let's delve into some of the key applications of AI across different fields.
AI in Healthcare
In the healthcare sector, artificial intelligence is more than just a buzzword; it’s a lifeline. AI tools are now employed for diagnostics, treatment personalization, and the improving of patient outcomes. For instance, algorithms analyze diagnostic images such as X-rays and MRIs, often detecting abnormalities faster than trained radiologists. This can be crucial for early detection of conditions, which often leads to better treatment.
"In a world where efficiency can save lives, AI becomes an indispensable ally for healthcare professionals."
Moreover, AI powers virtual health assistants, enabling patients to receive medical advice quickly. By leveraging machine learning, these virtual systems can help manage chronic diseases — providing tailored health recommendations based on accumulated data. The possibilities are immense and evolving, ensuring a healthier future.
AI in Finance
Finance absorbs AI much like a sponge. With the vast amounts of data that flood in daily, the need for intelligent systems that can sift through information is paramount. AI facilitates everything from automation of trading to risk assessment. For example, robo-advisors use algorithms to create and manage investment portfolios based on user risk preferences and market conditions.
Furthermore, fraud detection systems utilize AI to analyze transaction patterns and flag anomalies which could indicate nefarious activity. Banks and financial institutions benefit tremendously from this, saving both time and substantial amounts of money, as they can act on fraud alerts without the need for human intervention.
AI in Transportation
Transportation is another field that sees significant enhancements through AI. Autonomous vehicles, although still a work-in-progress, are an exciting application that promises to reshape how we view mobility. Companies like Waymo are at the forefront, developing self-driving technology that uses real-time data to navigate safely and efficiently.
Additionally, AI optimizes traffic management systems, predicting congestion and rerouting traffic dynamically. Public transport systems utilize AI to improve service efficiency, ensuring more reliable schedules and reducing wait times for users. All of these advancements intransportation hint at a future where AI not only makes travel safer but also more convenient.
AI in Customer Service
Customer service has undergone a transformation with the integration of AI solutions. Chatbots and virtual assistants respond to queries around the clock, ensuring that customer concerns are addressed promptly. This not only enhances customer satisfaction but also allows human agents to focus on more complex issues.
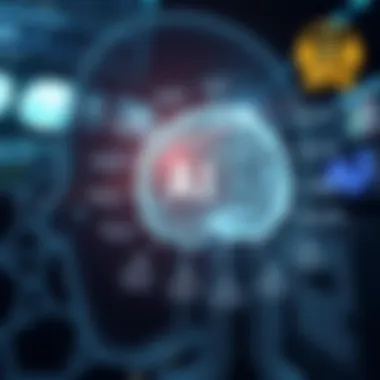
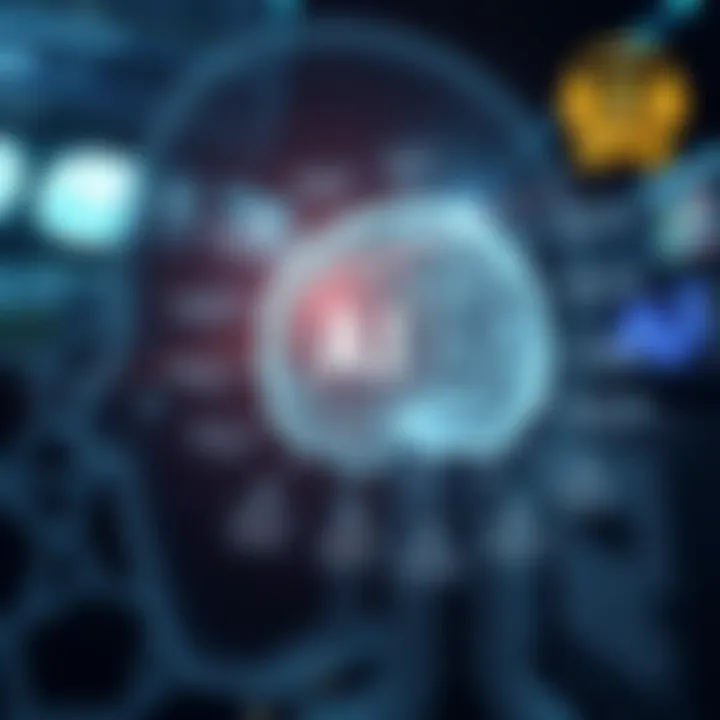
Often, businesses utilize AI to analyze customer interactions, gathering insights into consumer behavior and preferences. This data contributes to the creation of highly tailored marketing campaigns, resulting in improved customer engagement and sales.
AI has proven to be an invaluable resource across these domains, demonstrating its ability to innovate and streamline processes, ultimately leading to better outcomes. In summary, the embrace of artificial intelligence represents not just a technological evolution but a societal milestone that heralds significant change.
Ethical Considerations in AI
In today's fast-paced technological landscape, ethical considerations in AI are more critical than ever. As artificial intelligence systems become increasingly integrated into various sectors—be it healthcare, finance, or transportation—the ripple effects of their decision-making processes raise vital questions regarding ethics. Addressing these concerns is not just a matter of compliance, but a fundamental aspect of building trust and ensuring that AI serves humanity's best interests. Ethical considerations help highlight the balance between innovation and the potential social implications of AI technologies.
Bias and Fairness
One major ethical concern surrounding AI is bias. AI systems are often trained on large datasets that can contain historical biases, inadvertently perpetuating these issues in their outputs. For instance, a facial recognition system trained predominantly on images of lighter-skinned individuals may perform poorly when analyzing darker-skinned faces. The consequences of such biases can be detrimental, leading to unfair treatment in crucial areas like hiring processes or criminal justice.
To tackle these biases, developers must prioritize fairness during the design and training phases of AI. Techniques such as diversifying data sources and employing bias-detection algorithms can be effective measures. More importantly, fostering an inclusive team with diverse perspectives can help identify and mitigate bias from the outset.
"Fairness is not merely an absence of bias; it necessitates a holistic approach to creating equitable opportunities for all."
Privacy Concerns
Another pressing issue is privacy. With AI systems processing vast amounts of personal data, the potential for unauthorized access and misuse is alarmingly high. This could range from using consumer data for targeted marketing without consent to more severe implications involving surveillance systems that invade personal privacy. The more robust the AI, the greater the data requirements—all treading a fine line between utility and infringement.
Adopting stringent data protection policies, such as GDPR compliance, can serve as a buffer. Transparency in how data is collected, stored, and used builds consumer trust. Moreover, technologies such as differential privacy can be integrated to analyze data without compromising individual privacy, providing a delicate balance between functionality and confidentiality.
The Future of Work with AI
AI's growing prevalence in the workplace brings a spectrum of implications—both positive and negative. On one hand, it can automate mundane tasks, enabling human workers to focus on more creative and strategic activities. Think of AI taking over data entry; this can free up valuable human resources for brainstorming sessions or innovation. However, on the flip side, such automation raises fears of job displacement. Industries must grapple with transitioning their workforce in ways that minimize disruption while amplifying opportunities for growth and re-skilling.
In the future, collaboration between humans and AI will likely become the norm. Businesses will need to adapt to this hybrid model by investing in training programs that equip employees with the skills necessary for thriving in an AI-augmented environment. Likewise, policies must be established to protect workers, ensuring that technological advances do not come at the expense of livelihoods. Furthermore, ensuring that all individuals have access to relevant education and training is essential for fostering an equitable future in an AI-driven world.
AI Ethics is not simply an afterthought but a foundational aspect that must be considered at every step of development and implementation. By addressing bias, privacy concerns, and the future of work, stakeholders can work towards an AI landscape that benefits everyone.
Ending
In wrapping up this exploration of artificial intelligence, it's essential to reflect on the critical takeaway from our journey. Artificial Intelligence is not just a buzzword; it encapsulates a shift in how we interact with technology and the world around us. As discussed, AI is deeply rooted in complex algorithms and vast amounts of data, yet its applications resonate across various sectors - from healthcare to finance, and beyond.
Recap of Key Points
To summarize, let’s revisit the salient points we covered:
- Definition and Scope: We defined AI simply as the simulation of human intelligence processes by machines, especially computer systems, highlighting its role in replicating tasks traditionally performed by humans.
- Core Principles: The basic tenets of machine learning and the vital role of data in training AI systems were underscored, noting how these principles drive advancements.
- Types of AI: We distinguished between narrow AI that performs specific tasks and general AI that aspires to replicate human cognitive functions, touching on the nuances of reactive machines and self-aware AI.
- Real-World Applications: Practical examples in healthcare, finance, transportation, and customer service illustrated the tangible benefits of AI today.
- Ethical Considerations: The challenges of bias, fairness, privacy, and the future of work were discussed, making it clear that while AI offers immense possibilities, it also demands careful navigation.
The world of AI is vast and dynamic, making it imperative for professionals across various domains to stay informed.
Future Directions in AI Research
Looking ahead, the horizon for AI research is both promising and intricate. Here are some potential directions:
- Advancements in Understanding Natural Language: With the likes of OpenAI and Google working relentlessly, the capability of machines to comprehend and generate human language will leap forward, enhancing communication tools and services.
- Ethics and Governance: As AI becomes more embedded in society, researchers will focus more on creating ethical guidelines and systems to ensure fairness in AI operations.
- AI in Everyday Life: Expect to see deeper integration of AI in daily activities, such as home automation and personalized learning experiences, fundamentally changing how we manage our routines.
- Enhanced Machine Learning Models: Innovations will likely involve developing more sophisticated machine learning models that can learn from less data and adapt faster to new inputs.
- Collaboration Between Humans and AI: The future will likely see a shift towards AI as a collaborator, assisting human experts rather than replacing them, fostering an environment where technology and human intellect coalesce for superior outcomes.